7 Lead Scoring Best Practices to boost your conversions
- Agustin Bignu
- Sep 4, 2024
- 4 min read
Lead scoring is a technique widely used in marketing and sales. With the rise of AI, this technology started to be used for this process. If you are a non-technical person, it can be a difficult path to travel. The intention of this article is to elaborate what are the 7 best practices you should consider when implementing a predictive Lead Scoring model, because not having the necessary technical knowledge about AI does not mean that you cannot use this technology.
1. Simplicity over complexity
It is easy to fall into the trap of creating overly complex models due to the false belief that they will be better than a simpler approach. However, simplicity often leads to more effective results. A simple and clear model is easier to implement, understand and refine. Instead of trying to include as many variables as possible, focus on the key factors that actually drive conversion. By keeping your model simple, you will find it easier to adjust and optimise it over time.
On the other hand, a simple model also facilitates better communication with your sales and marketing teams. When everyone understands how leads are being qualified, it's easier to align efforts and make sure the process works as expected.
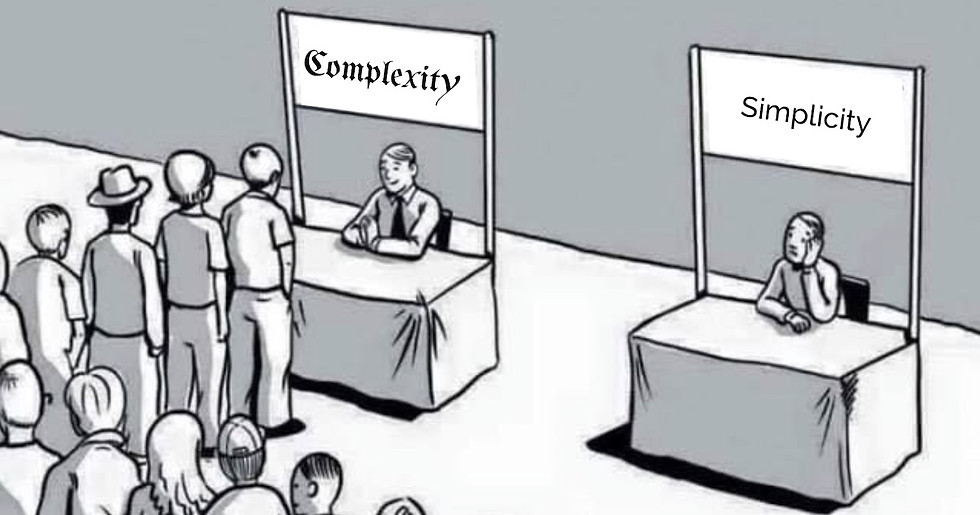
2. Quality over quantity
The quality of your data is far more important than the quantity. It is crucial to make sure that the data you use is accurate, well-organised and consistent. Incomplete or contradictory data leads to inaccurate scores, which ultimately hurts lead qualification. It pays to invest time in cleaning and validating your data before entering it into your model.
Prioritise data integrity to build a model that truly reflects the reality of your leads. Using multiple data sources will enrich your model, but make sure these sources are reliable and up-to-date. For example, combining CRM data with social media information can provide a more complete picture of a lead's potential.
3. Avoid personal data
One of the critical pitfalls in lead scoring is the use of personal data, as it can introduce bias into your model. Biases lead to unfair scores and can also expose your organisation to ethical and legal issues.
Instead, focus on behavioural data, such as the history of interaction with your website or interaction with emails, surveys, etc. This type of data is more indicative of a lead's intent and is less likely to introduce bias.
4. Focus on interpreting results
Interpreting results helps you understand the factors that drive lead scores to apply these insights to your sales funnel. AI models can produce complex outputs that might be difficult to understand without proper interpretation. This is where explainable or interpretable AI models come into play. These models are designed to provide information about how they arrive at their conclusions, making it easier to trust the results and act on them. This branch of study is known as explainable AI (X-AI).
In a hypothetical case, imagine the model indicates that leads who interact with certain types of content are more likely to convert, you can adjust your content strategy to target similar behaviours. Interpretation turns raw scores into actionable insights that drive better results.
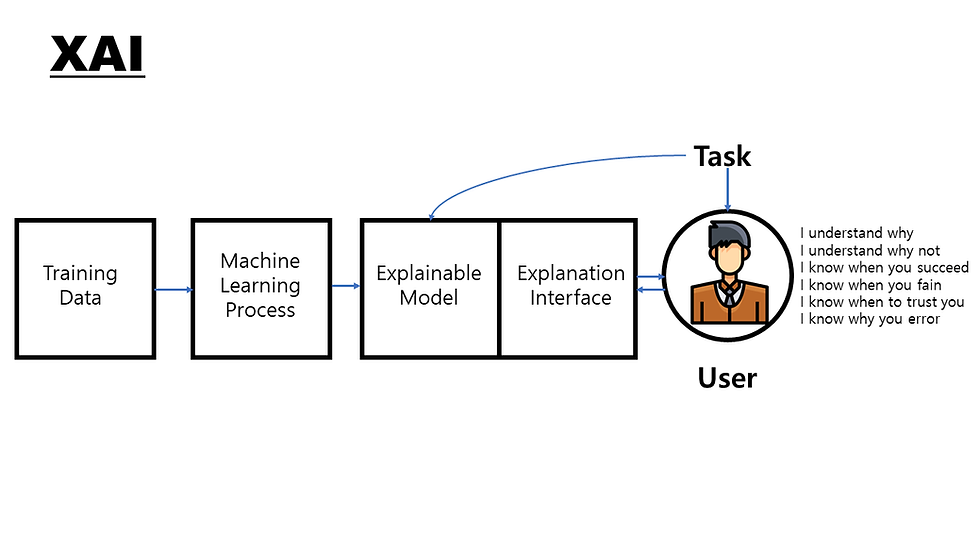
5. Create new rules and workflows
Your lead scoring model should not be a static tool; it should be a dynamic part of your sales strategy. Use the scores generated by your model to build new rules and workflows that optimise your funnel. You could create a rule that automatically assigns high-scoring leads to your top-performing sales reps. Alternatively, you could develop a workflow that triggers personalised email campaigns for leads with specific scores. The possibilities are endless.
6. Update the model regularly
AI models are not set-and-forget tools. To maintain effectiveness, your lead scoring model must be updated regularly to reflect new trends and data. If your model is based on outdated data, it will begin to produce irrelevant or inaccurate scores, leading to missed opportunities or wasted effort. Make it a priority to periodically re-train your model with the latest data to ensure it keeps performing well.
These updates also allow you to adapt to changes in your industry or target market. For example, if a new marketing channel becomes popular, your model should be updated to incorporate data from that channel.
7. Trust the process
AI-driven lead scoring is not a plug-and-play solution, but a long-term strategy that requires continuous attention and refinement. While it may take time to see significant results, the benefits of a well-honed model are worth it. As you collect more data and refine your approach, you will see increasingly accurate scores that translate into higher conversion rates.
Conclusions on Lead Scoring best practices
Make sure you focus on simplicity and quality, also avoid using personal data, and focus as well on getting interpretable results. Then adapt your model to make it a part of your sales process and keep it up-to-date in terms of data to keep up the rhythm of your sales calendar.
Remember, AI is a tool to aid decision-making, not to replace it. If you don't have a lead scoring algorithm in your sales process, you can try our Lead Scoring solution for free. Contact our team here.
Kommentare